In this blog post, I will be analyzing a loss-of-function splice variant in MTHFR using VarSeq. In the search for clinically relevant variants contributing to rare disorders, efficient filtering strategies are an important step in eliminating disinteresting variants. However, any applied filters must also ensure no interesting variants inadvertently get filtered out. Golden Helix provides the tools to complete this task with ease. VarSeq is the tertiary analysis tool whereby users can establish a clinical workflow to filter through their gene panel, exome, and genome-level data. In the clinical space, it is typical to construct panels to search for variants in genes with known links to the targeted disorder or phenotype. The purpose of this blog is to explore an example variant impacting the MTHFR gene (Methylenetetrahydrofolate Reductase) for a young patient diagnosed with cerebral folate deficiency (CFD).
The 5-month-old child began showing irritability and disturbed sleep. Eventually, symptoms progressed to ataxia which sparked the investigation into finding low cerebrospinal fluid 5-methyltetrahydrofolate in the nervous system. After reaching the diagnosis of CFD, a sample was processed through a next-gen sequencing pipeline to isolate any genomic component. The first stage of filtering in the tertiary analysis process was to filter variants to a targeted panel of a few genes tightly associated with CFD (Figure 1 & 2).

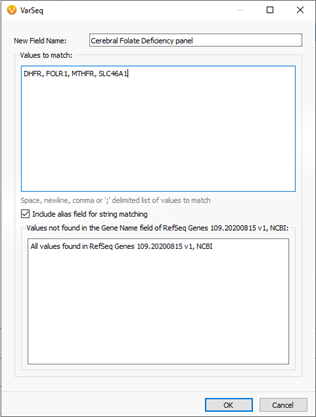
In addition to panel searches, users have a wide range of annotations to integrate into the workflow for additional filtering power. One may want to prioritize variants that lead to a missense or LOF effect. Filters such as these are available from the curated gene track RefSeq. You will see examples of filters in Figure 3 where users can select which sequence ontologies to prioritize, or those to remove. For example, users may wish to remove deep intronic variants. Additionally, you will find the simplified “Effect” filter to prioritize LOF or missense variants. In combination with the sequence ontology, it’s recommended to create splice site filters as well. This would include not only the prediction algorithms for GeneSplicer, MaxEnt Scan, Neural Network Splice, and Position Weight Matrix, but you can simply set the acceptable distance from the canonical splice site looking deeper into the intron. Using these filters in unison will ensure no interesting variant is missed.

Luckily, we did integrate splice site searching in our filter chain because we isolated a LOF splice donor variant in intron 10 (Figure 4). Moving through the variant evaluation process following the ACMG guidelines, we find that all splice algorithms agree for disruption (Figure 5). This is broken down into more detail in Figure 6, where you will find the score descriptions for each algorithm as well as the alternate allele placement in the genome sequence.



Processing all the variant guidelines in VSClinical we find that this variant is not only rare, but the splice variant is also creating a loss of function effect impacting protein. Additionally, looking through the literature search and submissions in ClinVar we discover that this gene is sensitive to LOF variants as there are other known pathogenic LOF variants reported. Ultimately, this variant was labeled as pathogenic and the clinician also discovered the relevance of LOF in MTHFR contributing to homocystinuria. This additional information was provided to the parents so as to assess diet and possibly limit the amount of protein intake.
This was meant to be a brief example of the value VarSeq provides in not only searching or filtering through massive genomic data but also the powerful application VSClinical in automating ACMG guidelines. There is much more to explore with this tool, and I would encourage you to reach out to our support team to schedule a call for a more thorough demonstration. Feel free to check out some of our other blogs that always contain important news and updates for the next-gen sequencing community.