Golden Helix software provides huge analytic gain in handling large-scale genomic data. For example, a number of VarSeq users run cohort projects of whole genome level data processing hundreds of millions of variants at a time. However, many of our users are running gene panel level data for custom panels related to cancer (both hereditary and somatic), autism, cardiac, and many other rare hereditary platforms. One major benefit of handling bioinformatic software support is gaining insight when troubleshooting user gene panel design. These panels can be targeted or untargeted which will influence the total number of variants that are carried through the tertiary analysis stage (i.e. filtering for clinically relevant variants). VarSeq is the tertiary solution that allows the users to integrate their custom panels to varying levels of strictness. The purpose of this blog will be to demonstrate available VarSeq features to build your preferred panel by exploring a TP53 missense variant relevant for medulloblastoma.
In this case, an 8 year old male patient suffered from motor delay, unsteady gait, and headaches. After inconclusive imaging results, clinicians sought to perform an NGS-based analysis. The goal was to isolate a variant responsible for the disorder associated with suspected brain tumor. The main issue here is the tricky diagnosis of medulloblastoma as opposed to general glioma. The solution is that when processing the data in VarSeq, users can specify multiple panel parameters if one filtering criteria isn’t quite achieving the goal.
For example, this sample was initially run through the following workflow (Figure 1).

Figure 1. Workflow logic concluded with panel-based search on Match Gene list linked to Phenotype
Stage 1. Eliminating low quality variants
Filtering based on VCF quality fields keeping variants as PASS, sufficient read depth (>=100), and sufficient genotype quality (>=20).
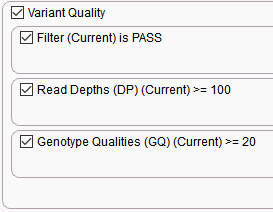
Stage 2. Removing well known benign variants seen in ClinVar
Inverted filtering logic (orange !) to remove benign/likely benign variants with high review status.

Stage 3. Removing common variants seen in germline population catalogs
Variant allele frequency filters from 1kg Phase 3 and gnomAD Genomes to keep rare (=<1%) and novel variants (missing).

Stage 4. Removing benign variants based on GHI integrated ACMG Classifier

Stage 5. Filtering variants based on Gene panel.
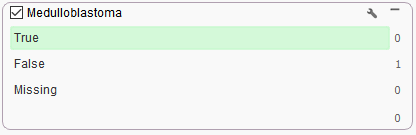
So, the final result of the filter is that there is a single variant as output, but unfortunately it lands outside of the medulloblastoma specific virtual panel using Match Genes Linked to Phenotypes (Figure 2). Fortunately, VarSeq has even more tools to leverage in this situation that can ensure all relevant variants are captured.
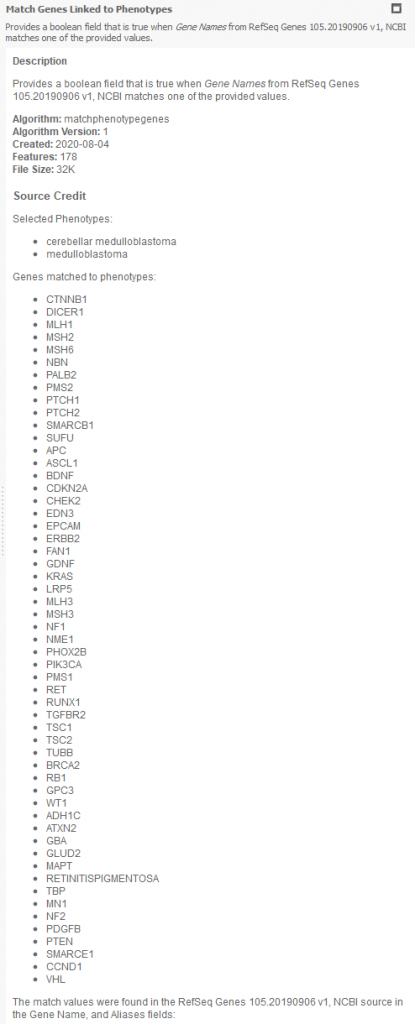
Figure 2. Example of Match Gene List linked to Phenotype search algorithm with +1 hop in Gene Ontology for medulloblastoma and cerebellar medulloblastoma.
One additional algorithm was then added to this workflow that leverages phenotypes and provides a ranked value of any variant association to that phenotype in relation to the gene. This algorithm is known as PhoRank and it was added to a filter container to add another dimension in the search of variants in genes related to specified phenotypes (Figure3). The PhoRank algorithm is based on Phevor but with search optimization to eliminate commonly linked genes not directly related to the phenotype. Overall, this tool is searching through the OMIM, Human Phenotype Ontology (HPO), and Gene Ontologies (GO), and generating a rank value of 0-1 for each variant. The benefit in this workflow is that the gene panel itself was a bit too specific, but PhoRank association of 90% or higher captured the TP53 missense variant associated with Medulloblastoma phenotypes.
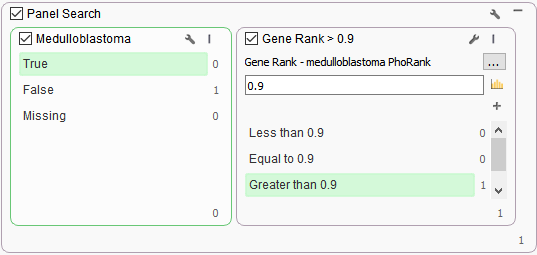
Figure 3. Two dimensional panel search for variants associated with Medulloblastoma using two algorithms, Match Gene List linked to Phenotypes and PhoRank.
Though the filter chain had many stages, users can think of the filtering process itself being step 1 of analysis, i.e. prioritizing the clinically relevant variant. Step 2 would be then to process the variant in the ACMG or AMP guidelines to determine pathogenicity/oncogenicity for the given variant. This TP53 missense variant is germline suspected so it will pass through the ACMG guidelines. This variant had a number of criteria ultimately providing enough weight to reach a final classification of pathogenic (Figure 4 ab). Reasoning being multiple submissions of pathogenic variants even known in ClinVar for brain tumors, predictions for conserved regions and damaging impact, and in hotspot regions for which TP53 is sensitive to missense variants.

Figure 4a. Details on filtered TP53 variant in VarSeq automated ACMG/AMP guideline tool VSClinical.

Figure 4b. Criteria associated with the filtered TP53 variant that add weight to final Pathogenic ACMG classification.
Unfortunately, there still needs to be more research done on this particular variants impact and TP53 relevance to medulloblastoma specifically. Medulloblastoma is known to hit males a bit harder than female patients but is nonetheless a devastating cancer. Reasons like this justify not only the need for high throughput capture of these relevant variants, but also increase the turnover of determining which treatment option combinations can be developed. Obviously there are many more details in analysis like this one and our support team at Golden Helix would be ecstatic about getting your panel/pipelines set up to process all your samples quickly but comprehensively. Please reach out to [email protected] to schedule a training or trial our software.
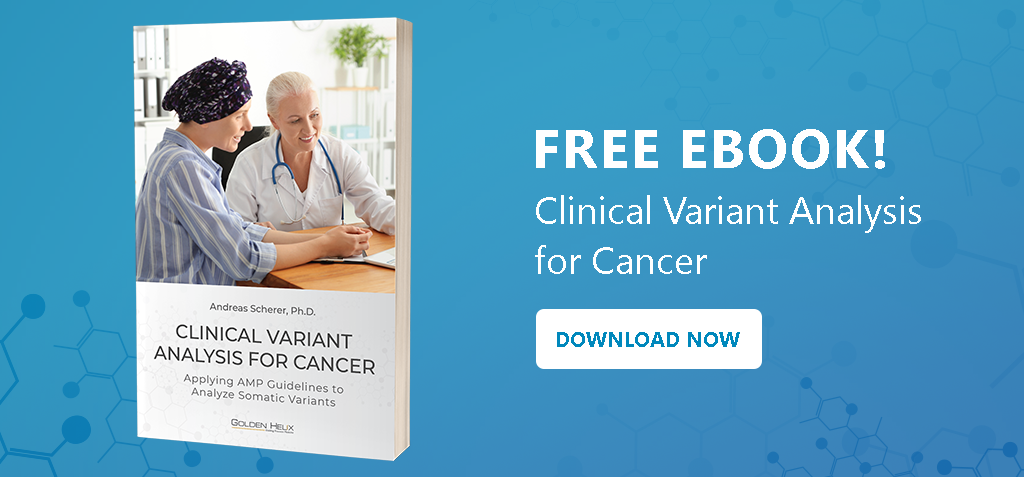